El Niño Southern Oscillation (ENSO) Phenomenon and the Analysis of Time Series Variability in Precipitation within the Guanacaste Conservation Area, Costa Rica
DOI:
https://doi.org/10.15359/rgac.72-18Keywords:
Guanacaste Conservation Area, precipitation, ecoregions, ENSOAbstract
The Pacific Northwest of Costa Rica is a region with marked seasonality in rainfall patterns. This area of Costa Rica is prone to extreme hydroclimatic phenomena such as droughts and floods. Due to the limited distribution of rainfall gauges and the unavailability of relevant information, complementary data obtained from satellites and their respective reanalyzes become imperative for acquiring crucial information. This information can support water resource management actions and their impacts on both natural and productive ecosystems.
To analyze the precipitation patterns, we utilized the CHIRPS product’s precipitation time series for five ecoregions within the Guanacaste Conservation Area, located in the northwestern Pacific region of Costa Rica. These curves were strongly and negatively correlated with time series from sea surface temperature monitoring regions, including Niño 1.2, Niño 3, Niño 3.4, and Niño 4.
All analyzed ecoregions exhibited strong negative correlations with the Niño 1.2 region, with correlation coefficients (R values) ranging between -0.72 to -0.74. Additionally, a lag of four to five months was observed in the Niño 4 curve compared to the Niño 1.2 region. This study suggests that the Niño 4 anomaly, with a lag of approximately 4 to 5 months, can serve as an indicator of possible impacts on precipitation patterns in different ecoregions. This provides sufficient time to plan actions, particularly within the agricultural sector. This study demonstrates the potential predictability of the effects of ENSO phenomenon on precipitation patterns for large areas with a certain eco-systemic homogeneity, such as the ecoregions in the Guanacaste Conservation Area.
References
Allen, K; Allen, K., Dupuy, J., Gei, M., Hulshof, C., Medvigy, D., Pizano, C, Salgado, B, et al. (2017). Will Seasonally Dry Tropical Forests Be Sensitive or Resistant to Future Changes in Rainfall Regimes? Environmental Research Letters, 12(2). https://doi.org/10.1088/1748-9326/aa5968.
Awange, J; Hu, K; Khaki, M. (2019). The Newly Merged Satellite Remotely Sensed, Gauge and Reanalysis-Based Multi-Source Weighted-Ensemble Precipitation: Evaluation over Australia and Africa (1981–2016). Science of the Total Environment, 670, 448–65.
Awange, J; Forootan, E. (2016). An Evaluation of High-Resolution Gridded Precipitation Products over Bhutan (1998–2012). International Journal of Climatology, 36(3), 1067–87.
Beck, H, Vergopolan, N; Pan, M; Levizzani, V; Van Dijk, A; Weedon, P; Brocca, P; Pappenberger, F; Huffman, G and Wood, E. (2017). Global-Scale Evaluation of 22 Precipitation Datasets Using Gauge Observations and Hydrological Modeling. Hydrology and Earth System Sciences, 21(12), 6201–17.
Cleveland, R; Cleveland, J; Terpenning, I. (1990). A Seasonal-Trend Decomposition Procedure Based on Loess (with Discussion). Journal of Official Statistics, 6(1).
Comité Regional de Recursos Hidráulicos CRRH y Sistema de la Integración Centroamericana SICA. (2019). El Niño Oscilación Del Sur (Enos). CRRH-SICA https://centroclima.org/wp-content/uploads/2019/02/El_Ni%C3%B1o_febrero_2019.pdf.
Corlett, R. (2016). The Impacts of Droughts in Tropical Forests. Trends in Plant Science, 21(7), 584–93. https://doi.org/https://doi.org/10.1016/j.tplants.2016.02.003.
Dee, P; Uppala, S; Simmons, A; Berrisford, P; Poli, P; Kobayashi, S; Andrae, U; et al. (2011). The Era-Interim Reanalysis: Configuration and Performance of the Data Assimilation System. Quarterly Journal of the Royal Meteorological Society, 137(656), 553–97.
Dinku, T., Funk, C., Peterson, P., Maidment, R., Tadesse, T., Gadain, H., & Ceccato, P2018). Validation of the Chirps Satellite Rainfall Estimates over Eastern Africa. Quarterly Journal of the Royal Meteorological Society, 144, 292–312.
Evans, J. (1996). Straight Forward Statistics for the Behavioral Sciences. Brooks/Cole Pub. Co, Pacific Grove.
Funk, C., Peterson, P; Landsfeld, P; Pedreros, D Verdin, J; Rowland, B; Romero, Husak, G; Michaelsen, J; and Verdin, A (2015). A Quasi-Global Precipitation Time Series for Drought Monitoring: U.S. Geological Survey Data Serie,s 832. https://doi.org/https://doi.org/10.1038/sdata.2015.66.
.Gorelick, N, Hancher, M; Dixon, M; Ilyushchenko, S; Thau, D; and Moore, R (2017). Google Earth Engine: Planetary-scale Geospatial Analysis for Everyone. Remote Sensing of Environment. https://doi.org/https://doi.org/10.1016/j.rse.2017.06.031.
Harris, I., Jones, P. D., Osborn, T., & Lister, D. (2014). Updated High-Resolution Grids of Monthly Climatic Observations–the Cru Ts3. 10 Dataset. International Journal of Climatologyt, 34(3), 623–642.
Hersbach, H., Bell, B., Berrisford, P., Hirahara, S., Horányi, A., Muñoz‐Sabater, Jet al. (2020). The Era5 Global Reanalysis. Quarterly Journal of the Royal Meteorological Society, 146(730), 1999–2049.
Hsu, K; Gao, X; Sorooshian, S, and Gupta, H. (1997). Precipitation Estimation from Remotely Sensed Information Using Artificial Neural Networks. Journal of Applied Meteorology and Climatology, 36(9), 1176–90.
Huffman, G, Bolvin, D., Nelkin, E; Wolff, D., Adler, R., Gu, G., ... Stocker, E. (2007). The TRMM Multisatellite Precipitation Analysis (TMPA): Quasi-global, multiyear, combined-sensor precipitation estimates at fine scales. Journal of Hydrometeorology, 8(1), 38-55.
Kidd, C., Bauer, P., Turk, J., Huffman, G., Joyce, R., Hsu, K., & Braithwaite, D (2012). Intercomparison of High-Resolution Precipitation Products over Northwest Europe. Journal of Hydrometeorology, 13(1), 67–83.
Kummerow, C., Barnes, W., Kozu, T., Shiue, J., & Simpson, J. (1998). The Tropical Rainfall Measuring Mission (TRMM) Sensor Package. Journal of Atmospheric and Oceanic Technology, 15(3), 809–817.
Losada, T., Rodriguez-Fonseca, B., Mohino, E., Bader, J., Janicot, S., & Mechoso, C. R. (2012). Tropical SST and Sahel Rainfall: A Non-Stationary Relationship. Geophysical Research Letters, 39(12).Ministerio de Planificación-Ministerio de Agricultura y Ganadería. (2015). Plan General de la Emergencia por Sequia. Según Decreto Ejecutivo N° 38642-MP-MAG, publicado en La Gaceta N°195, del viernes 10 de octubre del 2014. San José, Costa Rica. CNE. MP-MAG. https://www.pgrweb.go.cr/scij/Busqueda/Normativa/Normas/nrm_texto_completo.aspx?param1=NRTC&nValor1=1&nValor2=78091&nValor3=98281&strTipM=TC#ddown.
NASA. (2000). Digital Elevation Model - SRTM 1 Arc-Second 30m. NASA, NGA. http://lpdaac.usgs.gov
National Centers for Environmental Information. (s.f). Equatorial Pacific Sea Surface Temperatures. https://www.ncei.noaa.gov/access/monitoring/enso/sst.
National Weather Service, Climate Prediction Center (sf). Monthly Atmospheric & SST Indices. http://www.cpc.ncep.noaa.gov/data/indices/sstoi.indices.
Olson, D; Dinerstein, E. (2002). The Global 200: Priority Ecoregions for Global Conservation. http://maps.tnc.org/gis_data.html.
Personal del Área de Conservación Guanacaste. (2022). Sitio Web Del área de Conservación Guanacaste.
Pfeifer, M., Gonsamo, A., Woodgate, W., Cayuela, L., Marshall, A. R., Ledo, A.,,Paine, P; Marchant, P; et al. (2018). Tropical Forest Canopies and Their Relationships with Climate and Disturbance: Results from a Global Dataset of Consistent Field-Based Measurements. Forest Ecosystems, 5. https://doi.org/10.1186/s40663-017-0118-7.
Powers, J. S., Vargas G, G., Brodribb, T. J., Schwartz, N. B., Pérez‐Aviles, D., Smith‐Martin, C. M., ... & Medvigy, D. (2020). A Catastrophic Tropical Drought Kills Hydraulically Vulnerable Tree Species. Global Change Biology, 26(5), 3122-3133. https://doi.org/10.1111/gcb.15037
Qin, Y., Chen, Z., Shen, Y., Zhang, S., & Shi, R. (2014). Evaluation of Satellite Rainfall Estimates over the Chinese Mainland. Remote Sensing, 6(11), 11649–72.R Core Team. (2021). R: A Language and Environment for Statistical Computing. Vienna, Austria: R Foundation for Statistical Computing. https://www.R-project.org/.
Schneider, U., Becker, A., Finger, P., Meyer-Christoffer, A., Ziese, M., & Rudolf, B. (2014). GPCC’s New Land Surface Precipitation Climatology Based on Quality-Controlled in Situ Data and Its Role in Quantifying the Global Water Cycle. Theoretical and Applied Climatology, 115(1), 15–40.
Serrat, A; Valdes, J; Stakhiv, E. (2014). Water Management Applications for Satellite Precipitation Products: Synthesis and Recommendations. JAWRA Journal of the American Water Resources Association, 50(2), 509–25.
Sorooshian, S., Hsu, K;., Gao, X., Gupta, H., Imam, B., & Braithwaite, D. (2000). Evaluation of Persiann System Satellite-Based Estimates of Tropical Rainfall. Bulletin of the American Meteorological Society, 81(9), 2035–46.Tang,
L., Tian, Y., Yan, F., & Habib, E (2015). An Improved Procedure for the Validation of Satellite-Based Precipitation Estimates. Atmospheric Research, 163, 61–73.
Trenberth, K. (1997). The Definition of El Niño. Bulletin of the American Meteorological Society, 78(12), 2771–7. http://www.cgd.ucar.edu/staff/trenbert/trenberth.papers/defnBAMS.pdf.
Wang, B., Liu, J., Kim, H. J., Webster, P. J., Yim, S. Y., & Xiang, B (2013). Northern Hemisphere Summer Monsoon Intensified by Mega-El Nino/Southern Oscillation and Atlantic Multidecadal Oscillation. Proceedings of the National Academy of Sciences of the United States of America, 110(14), 5347–5352. https://doi.org/10.1073/pnas.1219405110Wilks, D. (2011). Statistical Methods in the Atmospheric Sciences. Academic press.
Wu, W., Li, Y., Luo, X., Zhang, Y., Ji, X., & Li, X (2019). Performance Evaluation of the Chirps Precipitation Dataset and Its Utility in Drought Monitoring over Yunnan Province, China. Geomatics, Natural Hazards and Risk, 10(1), 2145–62.
Yan, G; Yi, L; Xi, C. (2018). Evaluating Satellite-Based Precipitation Products in Monitoring Drought Events in Southwest China. International Journal of Remote Sensing, 39(10), 3186–3214.
Yun, K; Lee, J. Timmermann, A; and et al. (2021). Increasing Enso–Rainfall Variability Due to Changes in Future Tropical Temperature–Rainfall Relationship. Communications Earth & Environment. https://doi.org/10.1038/s43247-021-00108-8.
Zambrano, M. (2020). HydroTSM: Time Series Management, Analysis and Interpolation for Hydrological Modelling. https://github.com/hzambran/hydroTSM.
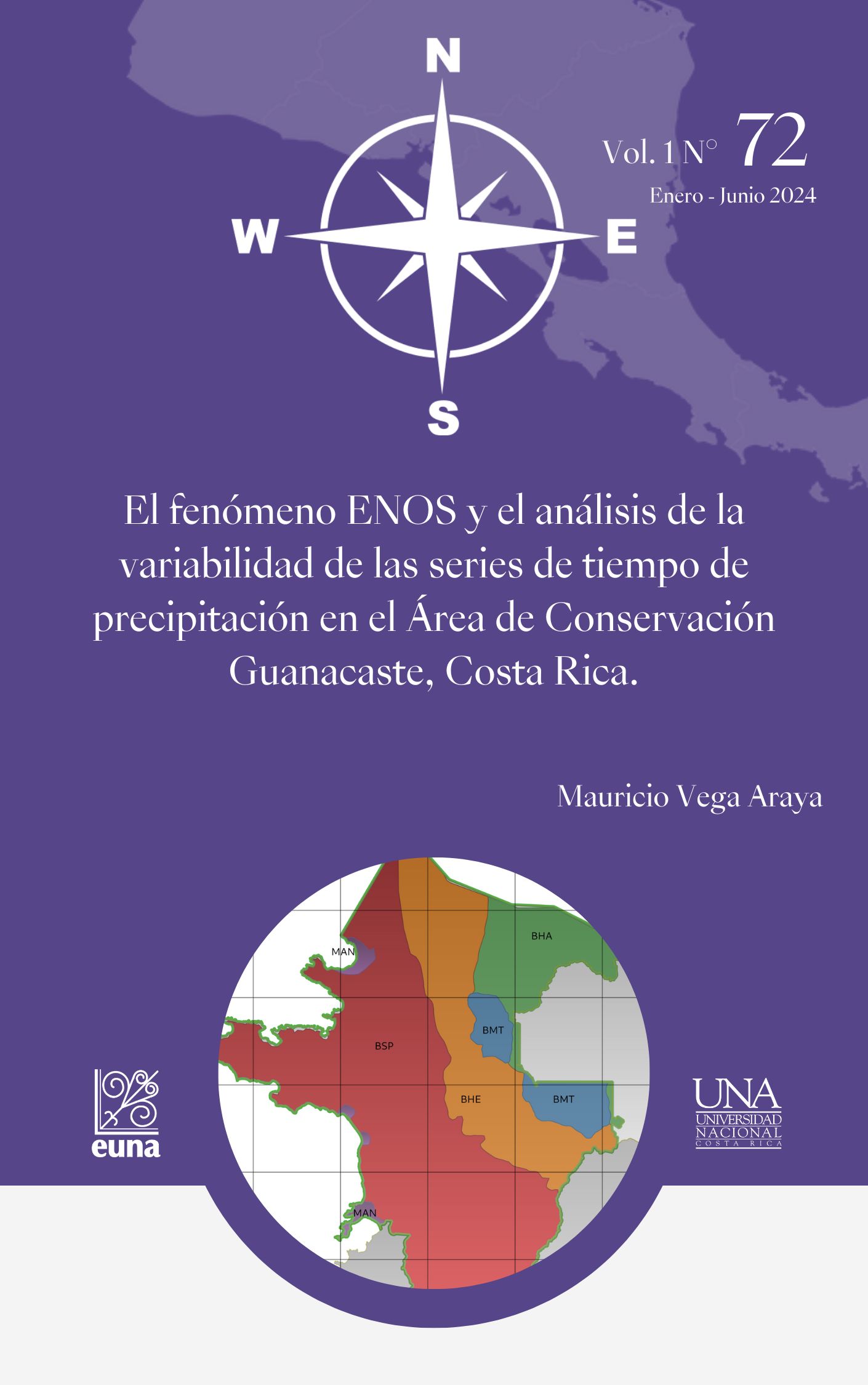
Published
How to Cite
Issue
Section
License
Copyright (c) 2024 Mauricio Vega-Araya

This work is licensed under a Creative Commons Attribution-NonCommercial-ShareAlike 4.0 International License.
Proposed policy for journals offering Open Access
Authors publishing their works in the Journal acknowledge and agree to the following terms:
a) Authors retain the copyrights to their works and guarantee the Journal the right to be the first to publish their works, under the Creative Commons License Attribution-NonCommercial-ShareAlike 4.0 International, CC BY-NC-SA 4.0 International (https://creativecommons.org/licenses/by-nc-sa/4.0/deed.es), which allows others to share works upon complying with the acknowledgment of authorship and mention of the Journal as the original publisher of the work.
b) Authors are permitted to separately establish additional agreements for the non-exclusive distribution of the official edition of the work published in the Journal (for example, authors may desire to place the work in an institutional repository or incorporate it into a book that is to published elsewhere) so long they acknowledgment to recognize the Journal as the original publisher. The aforementioned additional agreements must respect the terms of the non-profit character and sharing philosophy of the original license (CC BY-NC-SA 4.0 International, https://creativecommons.org/licenses/by-nc-sa/4.0/deed.es).
c) Authors are encouraged to archive the post-print or editor/PDF version in Open Access repositories.